Predicting Power Equipment Failure With Data Science
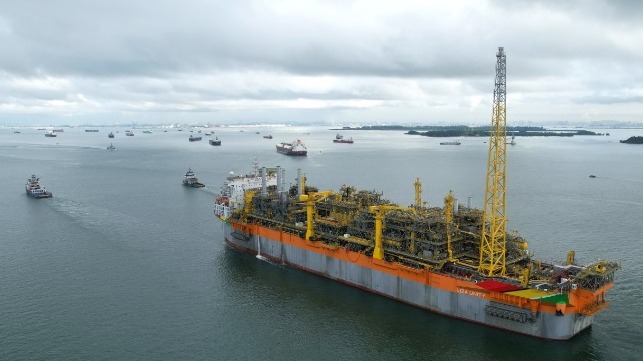
We all know that damaged and stranded ships can carry enormous safety and cost implications. According to the latest Safety and Shipping Review from Allianz Global Corporate & Specialty (AGCS), machine faults are the top cause of shipping incidents globally, accounting for four in every 10 during 2020 and creating serious safety concerns.
And of the 26,000-plus incidents over the past decade, more than a third (9,334) were caused by machinery damage or failure – over twice as many as the next highest cause (collision).
The financial cost can also be eye-watering. In the offshore oil and gas space, for example, organizations experience on average $49 million annually in financial impacts due to unplanned downtime, with the worst performers losing upwards of $88 million. On top of this, equipment failures can also create serious safety concerns.
Being able to perform anomaly detection to identify potential anomalous conditions, and from there predict failures ahead of time, can yield tremendous results in terms of operational continuity and decision making for maintenance, reducing operational costs and safety benefits to vessel operators and crew.
In addition to being able to detect anomalies and predict failures, accurate and reliable diagnoses are key to ensuring effective responses to problems. Whether this be in terms of inventory management, maintenance work or vessel operation to minimize the fault’s impact, data science can help decision makers to make the right calls at the right time.
Using data to keep ships switched on
Cases of machinery damage and failure can occur across a vast range of vessel equipment, including power components like propulsion diesel engines, ship service generators and their sub-components (mechanical or electrical). It is these power components which have formed the focus of ABS' work over the past two years.
The project has involved leveraging data from sensors that measure physical quantities such as currents, vibrations, temperatures and several other data points. Multiple statistical analysis and machine learning methods were adopted, helping to generate a factual understanding of key factors that affected equipment life and degradation.
We talk about this in terms of engine or machine operating cycles. In essence this is the amount of time between an engine being switched on and called on to produce power, to when being asked to shut down. For an electrical component, similarly, whenever it is asked to be turned-on the cycle commences until commanded to be shut off by the controller. This can last from a few minutes to a few hours, depending on the service factor for that particular piece of equipment.
Data from historical machine operating cycles was used to verify the strength of the relationships amongst the key factors. Here, we worked closely with domain experts to help us further explain some of the observations made.
Meanwhile, machine learning modeling was used to develop time-dependent models among the variables and factors affecting component life. The problem was to identify what may have caused a certain component to lose functionality, detect the initiation of these conditions and estimate how many remaining operating cycles might be left. This input would be vital for both ashore and onboard crew: decisions about inspection, maintenance planning, derating and operation profile changes could be taken with higher confidence.
Progress has been extremely promising to date, and we can now achieve an accuracy rate of greater than 90 percent over a prediction horizon of at least five operating cycles when it comes to predicting power component failure. This is critical – it means we can provide high confidence in component failure forecasting over a long period of time.
The implication this has for fleet owners and operators, along with their crews, is sizeable.
This data science technology and methodology is key to planning condition-based maintenance decisions such as parts procurement, tools and personnel planning, and maintenance scheduling. Condition-based maintenance has the potential to drastically reduce the costs associated with vessel downtime and number of accidents linked to faulty equipment.
Critically, the methodology can differentiate between specific failure modes for faster diagnostics, which can underpin decisions such as equipment service factor changes, onboard repairs carried out by crew or derating. It also allows crews to act immediately and appropriately – for instance, operating at reduced power to extend operational time before the component fault is fixed, thus reducing overall downtime. These decisions would minimize disruptions to vessel operations caused from unplanned downtime, allowing such operations to be extended until maintenance can be performed.
An additional important dynamic involves being able to detect whether a fault is due to natural degradation or the result of external factors such as cyberattacks.
Cybercrime is a growing problem for the marine industry. According to security specialist Naval Dome, as many as 300,000 maritime assets are now exposed to cyberattacks, with hacking incidents having grown by 400 percent during the first few months of the COVID-19 pandemic in 2020.
ABS’s data-driven methodology to predict and analyze power component failure has the potential to mitigate hacking damage by helping crew differentiate if such was the reason behind an observed fault.
A journey of continuous improvement
It is already being deployed in the real world, and as we seek to extend its rollout, our ambition is to safeguard more vessels from the damage that can be caused by power component faults, be they as a result of degradation or something more sinister.
This encompasses the work that ABS itself undertakes. The data science methodology provides vital inputs for conducting condition-based Class decisions, and enables us to carry out far more targeted, focused surveys which lead to reduced intervention and a better service for our clients.
Looking ahead, the project will continue to evolve and uncover further enhancements. Ultimately, this is an important step to optimizing condition-based maintenance operations and provisioning vessels crew with valuable autonomous decision support.
Meanwhile, we aim to initiate more collaborations between data scientists and various other stakeholders across the marine industry with a clear message: that using insights provided by data-driven programs can deliver enormous benefits across safety, OPEX, planning and decision-making, vessel availability, and more.
Indeed, we are confident we can leverage the power of data science to provide even greater certainty in power component failure predictions over longer time intervals – in doing so, the resultant gains to be made will be increasingly difficult to ignore.
Subrat Nanda is Chief Data Scientist for ABS.
The opinions expressed herein are the author's and not necessarily those of The Maritime Executive.