Winning an AI-Enabled War at Sea
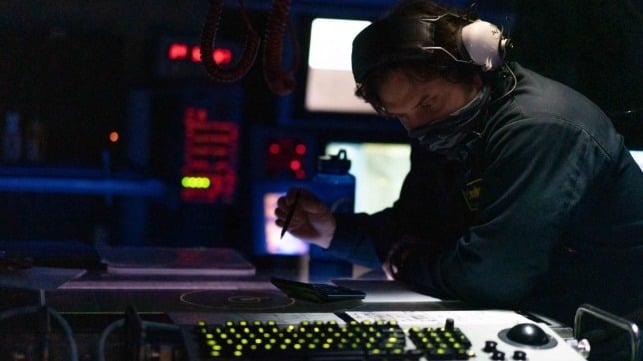
[By Dr. Peter Layton]
Artificial intelligence (AI) technology is suddenly important to military forces. Not yet an arms race, today’s competition is more in terms of an experimentation race with many AI systems being tested and new research centers established. There may be a considerable first-mover advantage to the country that first understands AI adequately enough to change its existing human-centered force structures and embrace AI warfighting.
In a new Joint Studies Paper, I explore sea, land and air operational concepts appropriate to fighting near-to-medium term future AI-enabled wars. With much of the underlying narrow AI technology already developed in the commercial sector, this is less of a speculative exercise than might be assumed. Moreover, the contemporary AI’s general-purpose nature means its initial employment will be within existing operational level constructs, not wholly new ones.
Here, the focus is the sea domain. The operational concepts mooted are simply meant to stimulate thought about the future and how to prepare for it. In being so aimed, the concepts are deliberately constrained; crucially they are not joint or combined. In all this, it is important to remember that AI enlivens other technologies. AI is not a stand-alone actor, rather it works in the combination with numerous other digital technologies. It provides a form of cognition to these.
AI Overview
In the near-to-medium term, AI’s principal attraction is its ability to quickly identify patterns and detect items hidden within very large data troves. The principal consequence of this is that AI will make it much easier to detect, localize and identity objects across the battlespace. Hiding will become increasingly difficult. However, AI is not perfect. It has well known problems in being able to be fooled, in being brittle, being unable to transfer knowledge gained in one task to another and being dependent on data.
AI’s warfighting principal utility then becomes ‘find and fool’. AI with its machine learning is excellent at finding items hidden within a high clutter background. In this role AI is better than humans and tremendously faster. On the other hand, AI can be fooled through various means. AI’s great finding capabilities lack robustness.
A broad generic overview is useful to set the scene. The ‘find’ starting point is placing a large number of low cost Internet of Things (IoT) sensors in the optimum land, sea, air, space and cyber locations in the areas across which hostile forces may transit. From these sensors, a deep understanding can be gained of the undersea terrain, sea conditions, physical environment and local virtual milieu. Having this background data accelerates AI’s detection of any changes and, in particular, of the movement of military forces across it.
The fixed and mobile IoT edge-computing sensors are connected into a robust cloud to reliably feed data back into remote command support systems. The command system’s well-trained AI could then very rapidly filter out the important information from the background clutter. Using this, AI can then forecast adversary actions and predict optimum own force employment and its combat effectiveness. Hostile forces geolocated by AI can, after approval by human commanders, be quickly engaged using indirect fire including long-range missiles. Such an approach can engage close or deep targets; the key issues being data on the targets and the availability of suitable range firepower. The result is that the defended area quickly becomes a no-go zone.
To support the ‘fool’ function, Uncrewed Vehicles (UV) could be deployed across the battlespace equipped with a variety of electronic systems suitable for the Counter Intelligence Surveillance And Reconnaissance And Targeting (C-ISRT) task. The intent is to defeat the adversary’s AI ‘find’ capabilities. Made mobile through AI, these UVs will be harder for an enemy to destroy than fixed jammers would be. Moreover, mobile UVs can be risked and sent close in to approaching hostile forces to maximize jamming effectiveness. Such vehicles could also play a key role in deception, creating a false and misleading impression of the battlefield to the adversary. Imagine a battlespace where there are a thousand ‘valid’ targets, only a few of which are real.
A War-at-Sea Defense Concept
Defense is the more difficult tactical problem during a war-at-sea. Its intent is solely to gain tactical time for an effective attack or counterattack. Wayne Hughes goes as far in his seminal work to declare that: “All fleet operations based on defensive tactics…are conceptually deficient.”1 The AI-enabled battlefield may soften this assertion.
Accurately determining where hostile ships are in the vast ocean battlefields has traditionally been difficult. A great constant of such reconnaissance is that there never seems to be enough. However, against this, a great trend since the early 20th century is that maritime surveillance and reconnaissance technology is steadily improving. The focus is now not on collecting information but on improving the processing of the large troves of surveillance and reconnaissance data collected. Finding the warship ‘needle’ in the sea ‘haystack’ is becoming easier.
The earlier generic ‘find’ concept envisaged a large distributed IoT sensor field. Such a concept is becoming possible in the maritime domain given AI and associated technology developments.
DARPA’s Ocean of Things (OoT) program aims to achieve maritime situational awareness over large ocean areas through deploying thousands of small, low-cost floats that form a distributed sensor network. Each smart float will have a suite of commercially available sensors to collect environmental and activity data; the later function involves automatically detecting, tracking and identifying nearby ships and – potentially – close aircraft traffic. The floats use edge processing with detection algorithms and then transmit the semi-processed data periodically via the Iridium satellite constellation to a cloud network for on-shore storage. AI machine learning then combs through this sparse data in real time to uncover hidden insights. The floats are environmentally friendly, have a life of around a year and in buys of 50,000 have a unit cost of about US$500 each. DARPA’s OoT shows what is feasible using AI.
In addition to floats, there are numerous other low-cost AI-enabled mobile devices that could noticeably expand maritime situational awareness including: the EMILY Hurricane Trackers, Ocean Aero Intelligent Autonomous Marine Vehicles, Seaglider Autonomous Underwater Vehicles, Liquid Robotics Wave Gliders and Australia’s Ocius Technology Bluebottles.
In addition to mobile low-cost autonomous devices plying the seas there is an increasing number of smallsats being launched by governments and commercial companies into low earth orbit to form large constellations. Most of these will use AI and edge computing; some will have sensors able to detect naval vessels visually or electronically.
All this data from new sources can be combined with that from the existing large array of traditional maritime surveillance systems. The latest system into service is the long-endurance MQ-4C Triton uncrewed aerial vehicle with detection capabilities able to be enhanced through retrofitting AI. The next advance may be the USN’s proposed 8000km range, AI-enabled Medium Unmanned Surface Vessel (MUSV) which could cruise autonomously at sea for two months with a surveillance payload.
With so many current and emerging maritime surveillance systems, the idea of a digital ocean is becoming practical. This concept envisages the data from thousands of persistent and mobile sensors being processed by AI, analyzed though machine learning and then fused into a detailed ocean-spanning three-dimensional comprehensive picture. Oceans remain large expanses making this a difficult challenge. However, a detailed near-real time digital model of smaller spaces such as enclosed waters like the South China Sea, national littoral zones or limited ocean areas of specific import appears practical using current and near-term technology.
Being able to create a digital ocean model may prove revolutionary. William Williamson of the USN Naval Postgraduate School declares: “On the ‘observable ocean’, the Navy must assume that every combatant will be trackable, with position updates occurring many times per day. …the Navy will have lost the advantages of invisibility, uncertainty, and surprise. …Vessels will be observable in port…[with] the time of departure known to within hours or even minutes. This is true for submarines as well as for surface ships.”3
This means that in a future major conflict, the default assessment by each warship’s captain might be that the adversary probably knows the ship’s location. Defense then moves from being “conceptually deficient” to being the foundation of all naval tactics in an AI-enabled battlespace. The emerging AI-enabled maritime surveillance system of systems will potentially radically change traditional war-at-sea thinking. The ‘attack effectively first’ mantra may need to be rewritten to ‘defend effectively first.’
The digital, ‘observable ocean’ will ensure warships are aware of approaching hostile warships and a consequent increasing risk of attack. In this addressing this, three broad alternative ways for the point defense of a naval task group might be considered.
Firstly, warships might cluster together, so as to concentrate their defensive capabilities and avoid any single ship being overwhelmed by a large multi-axis, multi-missile attack. In this, AI-enabled ship-borne radars and sensors will be able to better track incoming missiles amongst the background clutter. Moreover, AI-enabled command systems will be able to much more rapidly prioritize and undertake missile engagements. In addition, nearby AI-enabled uncrewed surface vessels may switch on active illuminator radars, allowing crewed surface combatants to receive reflections to create fire control-quality tracks. The speed and complexity of the attacks will probably mean that human-on-the-loop is the generally preferred AI-enabled ship weapon system control, switching to human-out-of-the-loop as numbers of incoming missiles rise or hypersonic missiles are faced.
Secondly, instead of clustering, warships might scatter so that an attack against one will not endanger others. Crucially, modern technology now allows dispersed ships to fight together as a single package. The ‘distributed lethality’ concept envisages distant warships sharing precise radar tracking data across a digital network, although there are issues of data latency that limit how far apart the ships sharing data for this purpose can be. An important driver of the ‘distributed lethality’ concept is to make adversary targeting more difficult. With the digital ocean, this driver may be becoming moot.
Thirdly, the defense in depth construct offers new potential through becoming AI-enabled, particularly when defending against submarines although the basic ideas also have value against surface warship threats. In areas submarines may transit through, stationary relocatable sensors like the USN’s Transformational Reliable Acoustic Path System could be employed backed up by unpowered, long endurance gliders towing passive arrays. These passive sonars would use automated target recognition algorithms supported by AI machine learning to identify specific underwater or surface contacts.
Closer to the friendly fleet, autonomous MUSVs could use low-frequency active variable depth sonars supplemented by medium-sized uncrewed underwater vehicles (UUV) with passive sonar arrays. Surface warships or the MUSVs could further deploy small UUVs carrying active multistatic acoustic coherent sensors already fielded in expendable sonobuoys. Warships could employ passive sonars to avoid counter-detection and take advantage of multistatic returns from the active variable depth sonars deployed by MUSVs.
Fool Function. The “digital ocean” significantly increases the importance of deception and confusion operations. This ‘fool’ function of AI may become as vital as the ‘find’ function, especially in the defense. In the war-at-sea, the multiple AI-enabled systems deployed across the battlespace offer numerous possibilities for fooling the adversary.
Deception involves reinforcing the perceptions or expectations of an adversary commander and then doing something else. In this, multiple false cues will need seeding as some clues will be missed by the adversary and having more than one will only add to the deception’s credibility. For example, a number of uncrewed surface vessels could set sail as the warship leaves port, all actively transmitting a noisy facsimile of the warships electronic or acoustic signature. The digital ocean may then suggest to the commander multiple identical warships are at sea, creating some uncertainty as to which is real or not.
In terms of confusion, the intent might be not to avoid detection as this might be very difficult but instead prevent an adversary from classifying vessels detected as warships or identifying them as a specific class of warship. This might be done using some of the large array of AI-enabled floaters, gliders, autonomous devices, underwater vehicles and uncrewed surface vessels to considerably confuse the digital ocean picture. The aim would be to change the empty oceans – or at least the operational area – into a seemingly crowded, cluttered, confusing environment where detecting and tracking the real sought-after warships was problematic and at best fleeting. If AI can find targets, AI can also obscure them.
A War-at-Sea Offense Concept
In a conflict where both sides are employing AI-enabled ‘fool’ systems, targeting adversary warships may become problematic. The ‘attack effectively first’ mantra may evolve to simply ‘attack effectively.’ Missiles that miss represent a significant loss of the task group’s or fleet’s net combat power, and take a considerable time to be replaced. Several alternatives may be viable.
In a coordinated attack, the offence might use a mix of crewed and uncrewed vessels. One option is to use three ship types: a large, well-defended crewed ship that carries considerable numbers of various types of long-range missiles but which remains remote to the high-threat areas; a smaller crewed warship pushed forward into the area where adversary ships are believed to be both for reconnaissance and to provide targeting for the larger ship’s long-range missiles; and an uncrewed stealthy ship operating still further forward in the highest risk area primarily collecting crucial time-sensitive intelligence and passing this back through the smaller crewed warship onto the larger ship in the rear.
The intermediate small crewed vessel can employ elevated or tethered systems and uncrewed communications relay vehicles to receive the information from the forward uncrewed vessel and act as a robust gateway to the fleet tactical grid using resilient communications systems and networks. Moreover, the intermediate smaller crewed vessel in being closer to the uncrewed vessel will be able to control it as the tactical situation requires and, if the context changes, adjust the uncrewed vessel’s mission.
This intermediate ship will probably also have small numbers of missiles available to use in extremis if the backward link to the larger missile ship fails. Assuming communications to all elements of the force will be available in all situations may be unwise. The group of three ships should be network enabled, not network dependent, and this could be achieved by allowing the intermediate ship to be capable of limited independent action.
The coordinated attack option is not a variant of the distributed lethality concept noted earlier. The data being passed from the stealthy uncrewed ship and the intermediate crewed vessel is targeting, not fire control, quality data. The coordinated attack option has only loose integration that is both less technically demanding and more appropriate to operations in an intense electronic warfare environment.
An alternative concept is to have a large crewed vessel at the center of a networked constellation of small and medium-sized uncrewed air, surface and subsurface systems. A large ship offers potential advantages in being able to incorporate advanced power generation to support emerging defensive systems like high energy lasers or rail guns. In this, the large crewed ship would need good survivability features, suitable defensive systems, an excellent command and control system to operate its multitude of diverse uncrewed systems and a high bandwidth communication system linking back to shore-based facilities and data storage services.
The crewed ship could employ mosaic warfare techniques to set up extended kinetic and non-kinetic kill webs through the uncrewed systems to reach the adversary warships. The ship’s combat power is not then in the crewed vessel but principally in its uncrewed systems with their varying levels of autonomy, AI application and edge computing.
The large ship and its associated constellation would effectively be a naval version of the Soviet reconnaissance-strike complex. An AI-enabled war at sea then might involve dueling constellations, each seeking relative advantage.
Conclusion
The AI-enabled battlespace creates a different war-at-sea. Most obvious are the autonomous systems and vessels made possible by AI and edge computing. The bigger change though may be to finally take the steady scouting improvements of the last 100 years or so to their final conclusion. The age of AI, machine learning, big data, IoT and cloud computing appear set to create the “observable ocean.” From combining these technologies, near-real digital models of the ocean environment can be made that highlight the man-made artefacts present.
The digital ocean means warships could become the prey as much as the hunters. Such a perspective brings a shift in thinking about what the capital ship of the future might be. A recent study noted: “Navy’s next capital ship will not be a ship. It will be the Network of Humans and Machines, the Navy’s new center of gravity, embodying a superior source of combat power.” Tomorrow’s capital ship looks set to be the human-machine teams operating on an AI-enabled battlefield.
Dr. Peter Layton is a Visiting Fellow at the Griffith Asia Institute, Griffith University and an Associate Fellow at the Royal United Services Institute. He has extensive aviation and defense experience and, for his work at the Pentagon on force structure matters, was awarded the US Secretary of Defense’s Exceptional Public Service Medal.
This article appears courtesy of CIMSEC and may be found in its original form here.
The opinions expressed herein are the author's and not necessarily those of The Maritime Executive.